Proposing empirical correlations and optimization of Nu and Sgen of nanofluids in channels and predicting them using artificial neural network
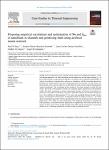
Ver/
Descargar
(application/pdf: 9.241Mb)
(application/pdf: 9.241Mb)
Fecha
2023-04-15Autor(es)
El Jery, Atef
Ramírez Coronel, Andrés Alexis
Orosco Gavilán, Juan Carlos
Al Ansari, Nadhir
Sh Sammen, Saad
Metadatos
Mostrar el registro completo del ítemResumen
Getting the best performance from a thermal system requires two fundamental analyses, energy
and entropy generation. An ideal mechanism has the highest Nu and the lowest entropy Sgen. As
part of this research, a large dataset of fluid flow via tubes has been collected experimentally. As
well as the inclusion of nanoparticles, analyses are included as well. By using deep learning algorithms, the Nusselt number and total entropy generation are predicted. In both models, the
mean absolute error was lower than 5%. To determine the most accurate model, hyperparameter
tuning is performed. That is adjusting all the settings in the neural network to attain the best
results. The results of the predictive models are compared against experimental and benchmark
results. The study incorporates a massive optimization strategy to fine-tune the predictive capabilities of the models. Furthermore, the model’s predictive abilities are evaluated through the
use of the coefficient of determination R2. For water and nanofluids flowing through circular,
square, and rectangular cross-sections, the proposed models can predict Nu and Sgen. The results
showed remarkable agreement with the experimental results. The models showed an MAE of not
higher than 1.33%, which is a great achievement. Also, empirical correlations are proposed for
both parameters, and double factorial optimization is implemented. The results showed that to
achieve the best results, the Re should be higher than 1600, and the nanoparticle concentration
should be 3%. A thorough justification of selected cases is presented as well.
Mostrar más
Cita bibliográfica
El Jery, A., Ramírez, A. A., Orosco, J. C., Al, N., & Sh Sammen, S. (2023). Proposing empirical correlations and optimization of Nu and Sgen of nanofluids in channels and predicting them using artificial neural network. Case Studies in Thermal Engineering, 45(2023), 102970. https://doi.org/10.1016/j.csite.2023.102970
Colecciones
El ítem tiene asociados los siguientes ficheros de licencia:
Excepto si se señala otra cosa, la licencia del ítem se describe como info:eu-repo/semantics/openAccess
Ítems relacionados
Mostrando ítems relacionados por Título, autor o materia.
-
La contabilidad ciencia predictiva: valoraciones y ventajas de su utilización
Zelada Alfaro, Wenceslao Fernando; Zelada Alfaro, Javier Enrique (Universidad Privada del Norte, 2003-06-01)Acceso abiertoRESUMEN La presente investigación formula la hipótesis que a partir de identificar los modelos científicos contables predictivos es posible valorar la función predictiva de la ciencia contabilidad, así como, es posible ... -
Mitigación de vibraciones mediante la simulación numérica directa (DNS), a campo lejano en una mina a tajo abierto - 2018
Díaz Espinoza, José Luis; Lucano Alvarado, Roger Sebastian (Universidad Privada del Norte, 2018-04-10)EmbargadoEn la actualidad en la industria de la minería en nuestro país, la voladura de roca es la técnica de extracción masiva más usada y que a su vez es una forma de generar vibraciones; por lo tanto, el conocimiento de su ... -
Desarrollo de una aplicación informática basada en un modelo de mahine learning para mejorar la evaluación de préstamos crediticios
Rodríguez Castillo, Jorge Junior; Miñano Ochoa, Milagros Madeleine (Universidad Privada del Norte, 2017-09-05)Acceso abiertoRESUMEN El presente trabajo de investigación está enfocado en el estudio de un modelo de machine learning para desarrollar una aplicación informática, que permita mejorar la evaluación de préstamos crediticios brindando ...